전체 글 썸네일형 리스트형 05. Convolutional Neural Networks In Lecture 5 we move from fully-connected neural networks to convolutional neural networks. We discuss some of the key historical milestones in the development of convolutional networks, including the perceptron, the neocognitron, LeNet, and AlexNet. We introduce convolution, pooling, and fully-connected layers which form the basis for modern convolutional networks. Keywords: Convolutional neura.. 더보기 04. Introduction to Neural Networks In Lecture 4 we progress from linear classifiers to fully-connected neural networks. We introduce the backpropagation algorithm for computing gradients and briefly discuss connections between artificial neural networks and biological neural networks. Keywords: Neural networks, computational graphs, backpropagation, activation functions, biological neurons slides Backpropagation Backprop is a rec.. 더보기 03. Loss Functions and Optimization Lecture 3 continues our discussion of linear classifiers. We introduce the idea of a loss function to quantify our unhappiness with a model’s predictions, and discuss two commonly used loss functions for image classification: the multiclass SVM loss and the multinomial logistic regression loss. We introduce the idea of regularization as a mechanism to fight overfitting, with weight decay as a co.. 더보기 02. Image Classification 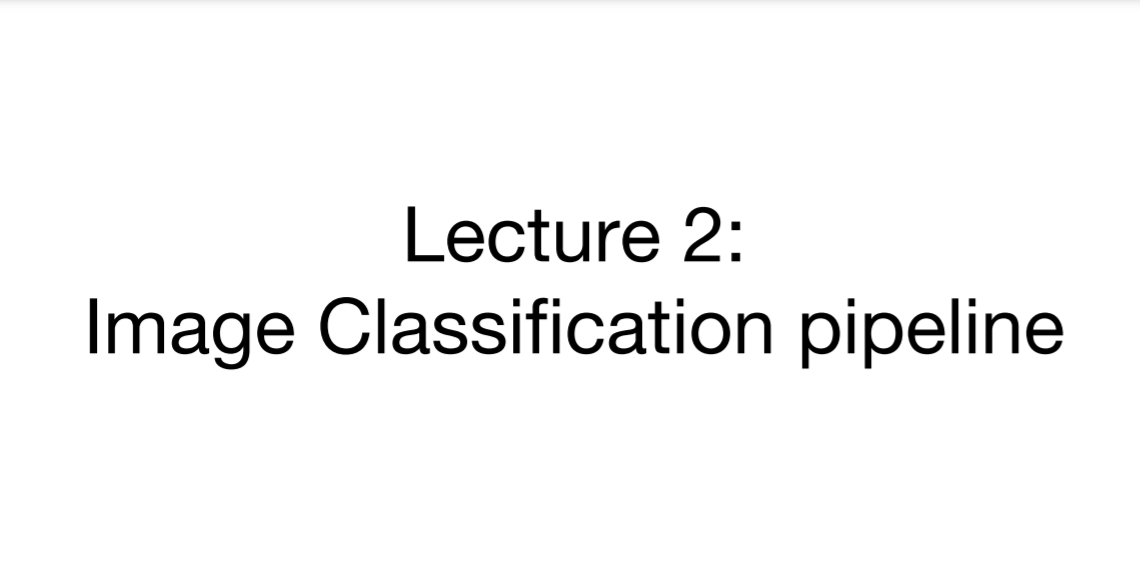 정리 카테고리 Lecture 2 formalizes the problem of image classification. We discuss the inherent difficulties of image classification, and introduce data-driven approaches. We discuss two simple data-driven image classification algorithms: K-Nearest Neighbors and Linear Classifiers, and introduce the concept.. 더보기 01. CS231n OT, Link link 더보기 [E-13] Bert 들어가며¶ BERT 논문 오늘은 BERT 모델 구조를 살펴보고, Pretrained Model을 활용하여 한국형 SQuAD인 KorQuAD task를 수행하는 모델을 학습해보자. 이 과정을 통해 Contextual Word Embedding의 개념과 자연어처리 분야의 최근 트렌드인 전이 학습 활용 방법까지 숙지해보자. 전제 조건 Keras를 활용한 모델 구성 및 학습 진행 방법을 숙지하고 있다. LSTM의 개념을 이해하고 모델 구성에 활용할 수 있다. Transformer 모델 구조와 Attention의 개념에 대해 이해하고 있다. 학습 목표 Transformer Encoder로 이루어진 BERT의 모델 구조를 이해한다. Pretrained embedding 접근 방식에 대해 이해한다. Pretraine.. 더보기 [E-12] Transformer, Chatbot In [2]: import tensorflow as tf import tensorflow_datasets as tfds import os import re import numpy as np import matplotlib.pyplot as plt print("슝=3") 슝=3 들어가며¶우리가 떠올리는 인공지능은 무엇인가? 인간 언어를 이해하고 인간과 자연어로 대화할 수 있는 기계를 우리는 자연스럽게 떠올리게 된다. 하지만 우리가 주변에서 흔히 보는 챗봇들이 모두 대화형인 것은 아니다. 챗봇의 5가지 대표 유형 링크를 보면 여러 챗봇들이 있다. 하지만 대화형 챗봇이 아니면 가지는 한계는 명확하다. 바로 사용자가 어떤 말을 하더라도 알아듣고 적절히 대응할 수 없다는 점이다. 챗봇과 딥러닝¶챗봇 역사의 모든 .. 더보기 [E-11] Stock prediction 들어가며 오늘은 시계열 예측을 다루는 여러 통계적 기법 중에 가장 널리 알려진 ARIMA(Auto-regressive Integrated Moving Average)에 대해 알아보고 이를 토대로 특정 주식 종목의 가격을 예측해보는 실습을 진행해보자. ARIMA는 탄탄한 통계학 이론적 기반을 갖추고 있으며, 시계열 데이터를 이해하는 관점을 훈련하는 데에도 도움이 된다는 점에서 한 번쯤 다뤄볼 만한 주제일 것이다. 학습 목표 시계열 데이터의 특성과 안정적(Stationary) 시계열의 개념을 이해한다. ARIMA 모델을 구성하는 AR, MA, Diffencing의 개념을 이해하고 간단한 시계열 데이터에 적용해 본다. 실제 주식 데이터에 ARIMA를 적용해서 예측 정확도를 확인해 본다. 목차 시계열 예측이란 .. 더보기 이전 1 ··· 4 5 6 7 8 9 10 ··· 13 다음