728x90
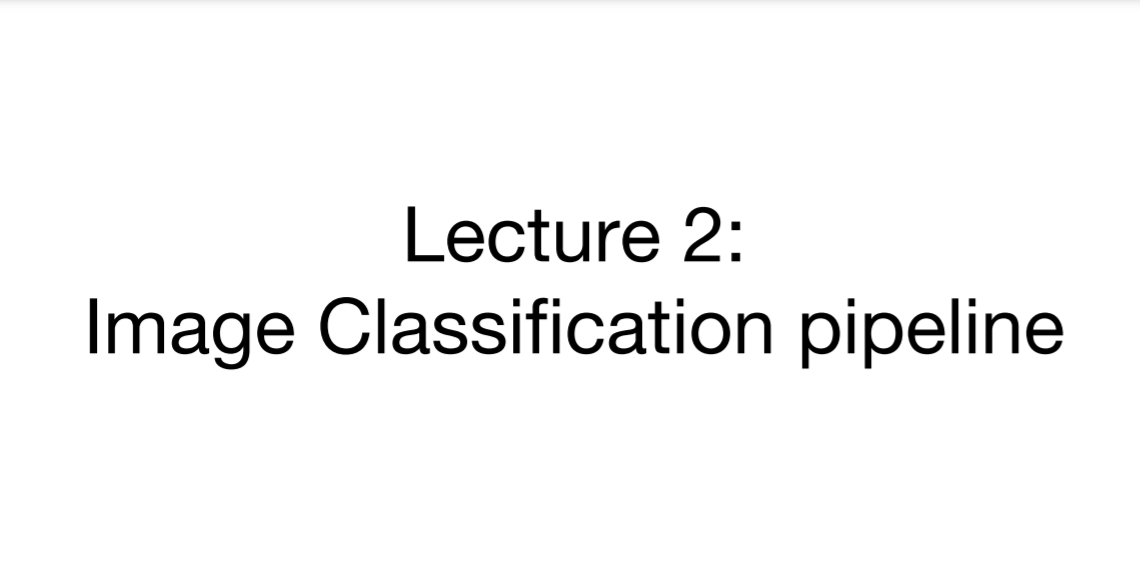
정리 카테고리
Lecture 2 formalizes the problem of image classification. We discuss the inherent difficulties of image classification, and introduce data-driven approaches. We discuss two simple data-driven image classification algorithms: K-Nearest Neighbors and Linear Classifiers, and introduce the concepts of hyperparameters and cross-validation.
Keywords: Image classification, K-Nearest Neighbor, distance metrics, hyperparameters, cross-validation, linear classifiers
slides:
http://cs231n.stanford.edu/slides/2017/cs231n_2017_lecture2.pdf
# How do we work on this image classification task?
- system input: images, predermined category labels
- what computer really seas: just a grid
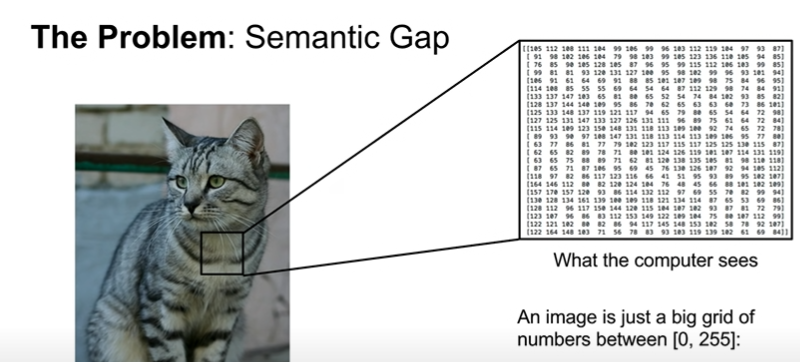
# Challenges:
our classification algorithm should be robust at different kinds of transforms.
1. Viewpoint variation
1. Illumination
1. Deformation
1. Occulusion
1. Background Clutter
1. Intraclasss variation
# Data-Driven Approach
1. Collect a datasset of images, labels
2. Use ML to train a classifier
3. Evaluate the classifier on new images
# Classifier
# K-Nearest Neighbor
- majority vote among K
- tends to smooth out our decision boundaries and lead to better results.
- L1 distance, L2 distance
- dependent on problem or data
- so just reccomend to try them both and see what works better
- Actually, never used
1. very slow at test time
2. Distance metrics on pixels are not informative
3. Curse of dimensionality
___
# Hyperparamets
- choices about the algorithm that we set rather than learn
## Setting Hyperparameters
1. Choose hyperparameters that work best on the data (Don't do this)
-> K=1 always works perfectly on training data
2. Split data into `train` and `test`; choose hyperparameters that work best on test data (Don't do this)
-> No idea how algorithm will perform on new data
3. Split data into `train` and `val`, and `test`; choose hyperparameters on val and evaluate on test (Better!)
4. Cross-Validation: Split data in folds, try each fold as validation and average the results
Q. training set vs validation set
- algorithm doesn't have direct accesss to the labels of `validation set.`
- uses validation set `only for checking` how well algorithm is doing.
728x90
'Computer Science > CS231n' 카테고리의 다른 글
06. Training Neural Networks(1) (0) | 2022.02.22 |
---|---|
05. Convolutional Neural Networks (0) | 2022.02.22 |
04. Introduction to Neural Networks (0) | 2022.02.22 |
03. Loss Functions and Optimization (0) | 2022.02.22 |
01. CS231n OT, Link (0) | 2022.02.22 |